Optimal operational efficiency & customer satisfaction thanks to artificial intelligence
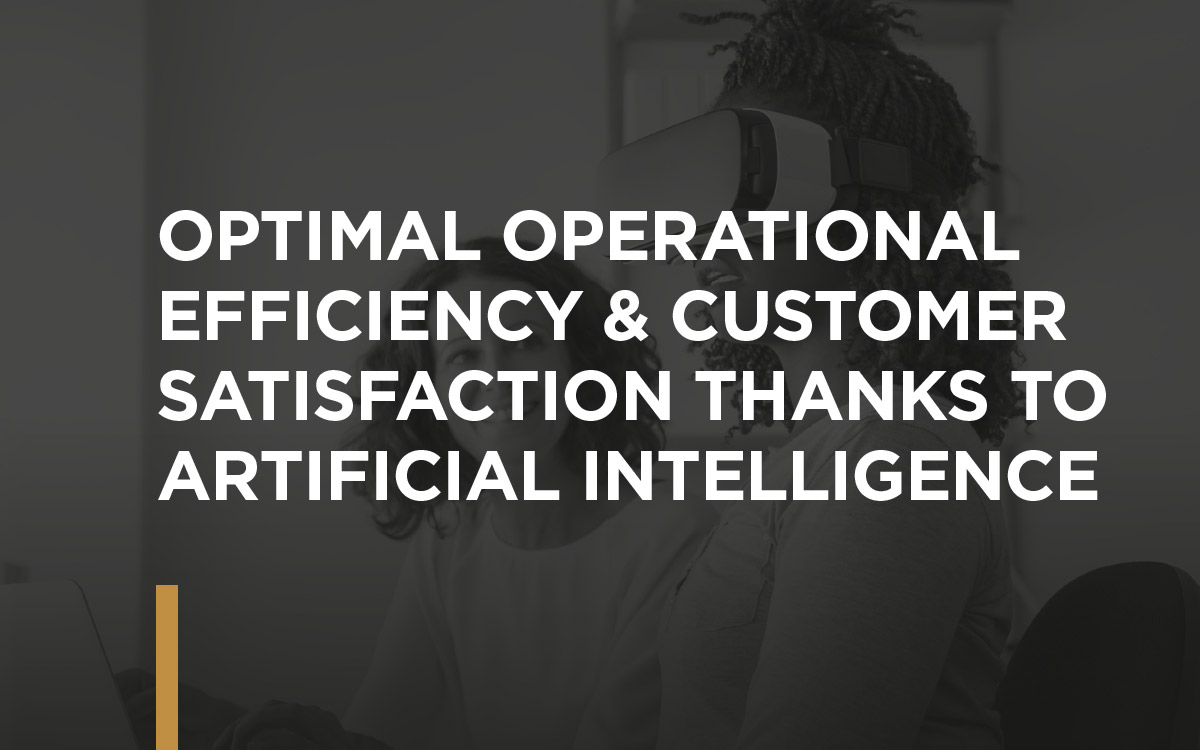
Square Management helped a major insurer to develop and deploy its data science & artificial intelligence strategy within its marketing department. The aim of this project, conducted at several levels, in particular data science and project management, was to implement data & AI tools and technologies at the heart of its management processes, particularly in order to streamline customer pathways and decision-making while ensuring improved customer satisfaction.
SOLUTIONS
1. Analysis of satisfaction & improvement of pathways
Several analytical approaches were set up to generalise the scientific study of customer pathways with regard to customer satisfaction:
- The first consisted in creating a data visualisation tool to measure the impact of customer pathways on satisfaction levels based on the analysis of claims information, contracts, customers, and customer/insurer interactions. The aim was not only to obtain a benchmark analytical tool regarding customer satisfaction, its evolution and its correlation with service level agreements (processing time, number of exchanges) but also to understand drivers of dissatisfaction.
- In addition to this data visualisation tool, the analysis of pathways with regard to satisfaction was reinforced by the use of a Process Mining tool, connected to the data in real time. By exposing all operational flows (communications, accounting operations, web browsing logs, etc.), it was possible to view all pathways and measure the impacts of all pathway deviations on a set of operational KPIs, including customer satisfaction. This large-scale project combined both technical challenges via the technological innovation represented by real-time connection (both for the insurer and the software publisher regarding the implementation and ingestion of a Kafka flow, the reference technology for processing data flows in real time) as well as operational challenges with regard to its ability to report management KPIs (in line with the steering already in force) and demonstrate their accuracy.
- Finally, in order to better understand the source of customer dissatisfaction, a Natural Language Processing project was set up to classify all verbatim customer reports. One of the major aims of this project was to detect actionable irritants for the organisation, by identifying:
- Blocking stages in the pathways,
- Discontent with service providers,
- The risk of customer attrition in the event of a strong threat.
- Etc.
These projects, the primary purpose of which was analytical, provided a starting point for data science use cases aimed at streamlining pathways and improving customer satisfaction. The use cases presented below describe in detail how Square supported this organisation in achieving these objectives, summarising for each project the methodological and technical issues encountered and their operational impacts.
2. Detecting fraud, promoting virtuous customers
One of the first high potential use cases identified was the creation of a compensation fraud detection engine for P&C and personal protection products. To succeed, this project had to reconcile several objectives:
- Explicability of the suspicion of fraud, a prerequisite expressed by claims managers;
- Effective identification of suspicious claims, striking a balance between high detection levels and a low volume of false positives;
- Availability, with detection as close as possible to real time.
To achieve the explicability objective, the decision was taken, together with the fraud detection teams, to create a set of rules to isolate suspicious behaviour and patterns. These rules made it possible to highlight the characteristics leading to the issuing of an alert. In addition, the decision was also taken to set up a feedback loop for these alerts, making it possible to measure their relevance and reliability, on the one hand, and build fully analysed and therefore labelled subsets of claims, on the other. This was designed to improve the detection process, both by improving the existing rules and also by enabling the implementation of a Machine Learning overlay, the aim of which was to prioritise alert levels.
While the artificial intelligence engine responded effectively to the challenges of optimising internal processes and reducing exposure to the risk of fraud, it did not help to improve customer satisfaction. In order to address both issues, we set up an identification engine for «trusted» customers: specifically, these were customers with a high «Customer Lifetime Value» (CLV), in order words who presented no risk of fraud and whose claims did not present any major financial exposure.
Operationally and from a customer point of view, this resulted in a reduced list of required supporting documents and, ultimately, a swifter compensation process. For the organisation, this made it possible to strengthen the loyalty of its best customers and focus its operational resources on high-stakes claims, while controlling its risk.
As a pioneering project in the big data environment of the marketing department, a significant effort was also made to obtain a 360° vision of the Data Lake on d+1, fed into directly from the management systems. In order to facilitate access to and understanding of the data by the data scientists and data analysts, an intermediate layer of data transfer from the various source systems was put in place, structuring the information by Data Vault modelling: this new data model had the advantage of being structured according to business objects (customers, claims, contracts, cover, accounting operations and communications) and of being multi-system and accessible to all data users.
3. Proactive management of customer relations
In order to meet the objective of preventing customer dissatisfaction without waiting for the business relationship to reach breaking point, our team of data scientists equipped the organisation with the capacity for realtime management of the risk of dissatisfaction, and by extension of attrition, thanks to the implementation of a proactive management project for the compensation process.
By cross-checking the exhaustive nature of customer compensation pathways with regard to satisfaction, it was possible to highlight those pathways with high degrees of dissatisfaction. By reinforcing this observation through a combination of several Machine Learning approaches (supervised model predicting dissatisfaction: decision tree, logistic regression), it was possible to create clusters of dissatisfaction, defined by:
- Claim characteristics,
- The types of service providers (repair shops) involved,
- Notions of time between management phases,
- The types of flows exchanged between the customer and the insurer.
Claims managers are now equipped to take the following appropriate actions to limit irritants from the customer’s point of view, transmitted in the form of alerts sent directly to the operational system:
- Request supporting documents,
- Send an expert a reminder,
- Trigger compensation,
- Inform the customer that their claim is in progress when no anomaly is observed,
- Etc.
As well as having a real impact on customer satisfaction, this approach also makes it possible to improve the organisation’s service levels by reducing the number of flows exchanged and shortening the time to compensation, thus providing a direct return on investment in addition to customer satisfaction.
4. Provider recommendation engine
As shown by an analysis of the tools for monitoring and classifying verbatim reports, the risk of customer dissatisfaction also derives from the customer’s relationship with the various service providers outside the establishment (experts, repair shops, etc.).
By capitalising on the knowledge acquired about these service providers through various projects, our team decided to intervene in the scope of automobile repair shops. In addition to the key issue regarding customer satisfaction, this scope was also prioritised in order to provide, simultaneously, a tool for monitoring and managing the performance of these service providers, particularly in order to set up special relationships with certain partners (or, on the contrary, to exclude certain partners) and to control costs.
As well as the customer’s location, therefore, this recommendation engine also took into account information regarding:
- The satisfaction generated by the garage,
- Its control of costs and deadlines,
- Its sustainability commitments.
In order to create this recommendation engine, our team had to deal with several issues, including access to information and modelling in an unsupervised context:
- With regard to satisfaction, the low volume of surveys risked this aspect not being representative; recourse to external sources was therefore necessary to obtain approximate satisfaction via the recommendation,
- In terms of costs, lead times and sustainability, no specific characteristic exists in principle to define the “best” repair shops (a better garage in terms of cost is not necessarily the one with the lowest average cost, which would automatically exclude those specialising in high-end products, for example).
Unsupervised classification algorithms (mixed classification and percentile clustering) by skills group and garage characteristics were therefore put in place to overcome this issue.
This recommendation engine, which is still in the process of being rolled out, nevertheless makes it possible to define new customer promises regarding quality of service levels and compliance with commitments. For the insurer, the aggregated view of garages’ performance offered by the scoring has already enabled the implementation of actions to clean up and streamline the service relationships.
Results
Thanks to the various projects carried out, the supporting role fulfilled by Square made it possible to initiate and consolidate the strategic development of the marketing department’s ambitions with regard to data science and artificial intelligence. By building trust through strong technical and business expertise, this support helped the organisation to achieve its transformation objectives and thus become more data-centric. Implementation of projects with the twofold objective of increasing operational efficiency and improving customer satisfaction was a major success for the organisation, enabling it to manage its costs, reduce risk and optimise internal processes, while ensuring the maximum satisfaction and therefore loyalty of its customers.
THE
SQUARE MANAGEMENT
-
Dual business and technical skills for an optimal vision of project management from an operational point of view
-
Detailed knowledge of insurance processes and in particular the customer pathway and the compensation process, enabling it to implement widespread use of AI in symbiosis with the operational teams
-
Particularly advanced technical expertise in data science to guarantee the robustness of the solutions deployed
-
Recognised expertise in data project management, particularly with regard to change management and the development of a data culture
CONTACT
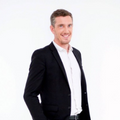
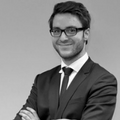